Density-functional theory (DFT) is by now recognized as the standard in theoretical condensed-matter studies, and the story of its successes can be found daily in the scientific literature.
DFT is quite efficient numerically, but it quickly runs into its limitations when the size of the system grows or when long-time simulations are required. To alleviate this problem, simpler models that are faster to evaluate are often used, at the price of reduced accuracy.
Here we present practical approaches to develop two such models, specifically density-functional tight-binding and neural-network based force fields, that are very efficient but that retain the precision of DFT in some situations. This is achieved by training the models to reproduce key quantities taken from a completely unbiased training set. We then present a few applications of these models, namely for the study of 2D atomic sheets, the melting of simple metals, etc.
Miguel Marques (Germany): The challenge of large systems: Tight-binding models and machine learning force-fields with the precision of density-functional theory
Location:
Verwandte Dateien
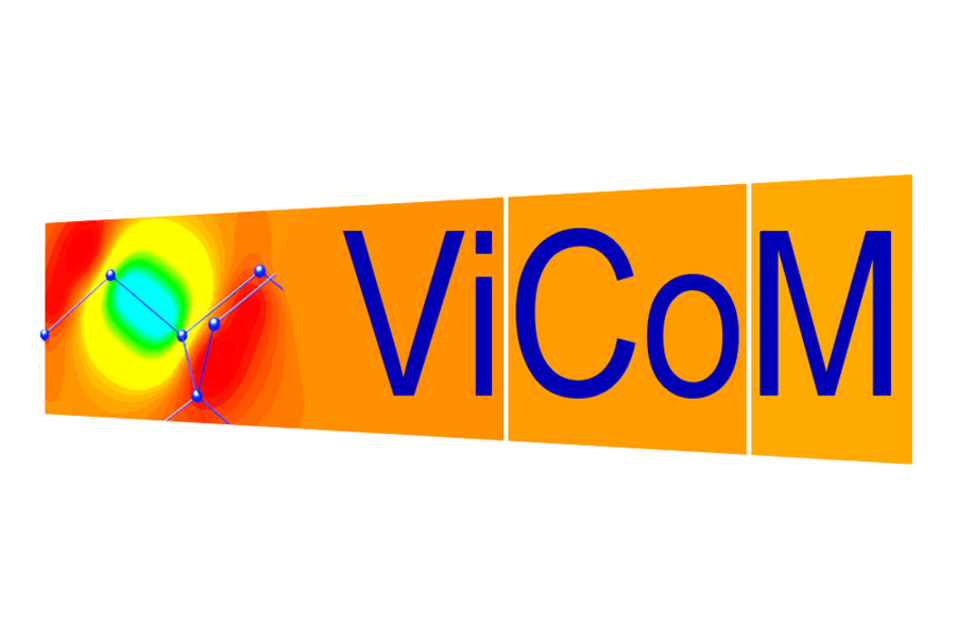